Meet Mark, a legal professional who needs to visit his dentist. Between his workloads, scheduling appointments feels like a big task. To make the situation worse, his last dental visit was frustrating. On top of that, he constantly worries about accurate diagnosis. But what if there was a way to streamline appointments, improve access to care, and even offer accurate diagnoses? Enter AI.
AI is already changing vast dynamics in healthcare, such as analyzing medical images and assisting doctors in catching potential issues earlier. In this blog, we will delve into the world of AI in healthcare, exploring how it’s overcoming several challenges and painting a future for a more efficient, personalized, and potentially life-changing healthcare delivery.
AI in the Driver’s Seat
The time that robots perform surgeries has passed by. The empowering capabilities of AI are driving real innovation in healthcare. Let’s discover how;
Meeting the Demands of an Aging Population
The aging population is one of the primary aspects driving AI integration in healthcare. As the population ages, there is a greater demand for efficient and effective healthcare solutions. However, AI offers extreme potential to address different challenges by streamlining processes, improving diagnostic accuracy, and facilitating personalized treatment plans. Healthcare professionals can optimize their resources and seamlessly provide better care for an ever-growing number of elderly patients.
Addressing Increasing Healthcare Costs
Another important factor fueling the need for AI in healthcare is the increasing costs of medical services. Every year, healthcare expenses continue to climb up, putting a strain on both patients and healthcare systems. AI solves these challenges by streamlining administrative processes, optimizing resource allocation, and reducing errors. Technology empowers healthcare professionals to improve patient outcomes and make healthcare affordable and accessible at the same time.
Growing Adoption of Digital Health
The healthcare industry is vastly embracing digital advancements in its offerings. Patients are also utilizing wearables, and telemedicine platforms are becoming more prevalent. AI plays an important role in this digital health ecosystem. It empowers healthcare providers to make data-driven decisions, analyze vast amounts of patient information efficiently, and provide personalized care remotely. Implementing AI within digital health is innovating and transforming how healthcare is delivered.
By observing these market forces, we can see that AI isn’t just a technological marvel; it’s a strategic solution to the evolving needs of the healthcare industry. It’s about efficiency, cost-effectiveness, and delivering better healthcare for a growing, technologically driven society.
Related read: What Every Healthcare CEO Should Know About Generative AI
Challenges of Commercializing AI in Healthcare
Bringing AI solutions to the healthcare industry comes with a lot of challenges. Here are some of the challenges we highlighted;
Data Privacy and Security
One of the important challenges to AI in healthcare is data privacy and security. As AI relies heavily on large amounts of patient data for analysis and decision-making, it is crucial to manage data security measures. Strong data encryption, secure storage systems, and strict access controls are crucial to maintain patient privacy and prevent unauthorized access or issues.
Regulatory Hurdles
Navigating the regulatory standards is another challenge for AI-powered medical devices and solutions. Clear guidelines and regulations are required to ensure the safety, efficacy, and ethical use of AI technologies in healthcare. Without clear guidelines, companies might hesitate to invest heavily in this space.
Cost and Investment
Developing, testing, and implementing AI in healthcare requires considerable financial resources, expertise, and infrastructure. AI development costs include data acquisition, algorithm development, training and validation, integration with existing systems, and ongoing maintenance. Healthcare organizations must carefully consider AI initiatives’ return on investment (ROI) and long-term sustainability.
Acceptance by Healthcare Professionals
The acceptance and adoption of AI technologies by healthcare providers present a unique challenge. There is a constant concern about AI replacing human jobs or making important decisions without human guidance. Building trust in AI among healthcare providers demands transparent communication, training, and collaboration. AI should be positioned as a tool to augment human capabilities, improve decision-making, and improve patient care rather than replace human expertise.
Start Your Generative AI Adventure Today! Unlock Creativity, Learn, and Create.
Strategies for Successful Commercialization
When it comes to commercializing AI in healthcare solutions, there are several key strategies that companies should consider. We have listed some strategies to help healthcare organizations ensure their solutions are successful in the market. Let’s delve deeper;
Address Clear Needs
To stand out in the market dynamics, companies must identify specific areas where AI can make a huge impact. They can focus on addressing clear needs and defining how their AI solutions can improve patient outcomes or streamline healthcare operations.
Collaboration with All Stakeholders
Collaboration is key to the successful implementation of AI in healthcare. Companies must understand the value of collaboration between AI developers, healthcare providers, regulators, and patients. By involving all stakeholders from the early stages of development, companies can ensure their solutions are aligned with the needs and requirements of the industry.
Transparency and Explainability
Transparency is crucial to building trust and acceptance in AI healthcare solutions. Companies must address the need for AI models to be transparent and explain their decision-making processes. Clear explanations of how the AI algorithms work and the factors influencing their decisions can reduce the concerns and build confidence among healthcare providers, regulators, and patients.
Focus on Data Quality and Security
Companies should highlight the importance of using higher quality and secured data to ensure accurate and reliable results. Strong data governance practices should be implemented to maintain data integrity while adhering to privacy regulations. By prioritizing data quality and security, companies can improve the effectiveness and trustworthiness of their AI healthcare solution.
How Does Mindbowser Help You Commercialize AI in Healthcare?
The journey of commercializing AI in healthcare is not just about technological advancements; it’s about creating a business ecosystem that establishes collaboration, addresses challenges, and delivers meaningful value to patients and healthcare providers.
As AI evolves in healthcare, businesses must address specific pain points and challenges healthcare providers face. Collaboration with stakeholders, including healthcare providers, regulators, and patients, is important to ensure alignment with regulatory requirements and user needs.
Moreover, prioritizing data quality and security is important to maintain integrity, reduce bias, and protect patient privacy. Strong data governance practices and adherence to regulatory standards are fundamental to AI’s success and ethical use in healthcare.
Experts at Mindbowser offer you a strategic approach encompassing technological innovation, collaboration, transparency, and data security. We embrace these principles and establish a strong business ecosystem that unlocks the full potential of AI to transform healthcare delivery, enhance patient outcomes, and drive sustainable growth in the healthcare industry.
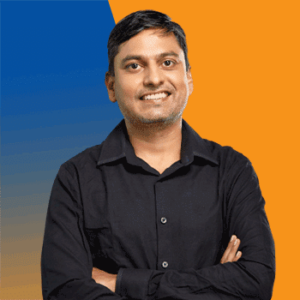
Sandeep Natoo
Head of Emerging Tech
Sandeep Natoo is a seasoned technology professional with a wealth of experience in software development, project management, and leadership. With a strong background in computer science and engineering, Sandeep has demonstrated exceptional proficiency in various domains of technology.
He is an expert in building Java-integrated web applications and Python data analysis stacks. He has been known for translating complex datasets into meaningful insights, and his passion lies in interpreting the data and providing valuable predictions with a good eye for detail.