Every field today has some level of AI integration. Even subsets of AI, such as Generative AI, are gaining traction daily. We live in a world where our morning news is personalized according to our interests with the help of AI.
According to a survey by the Pew Research Center, an astonishing 63% of individuals express deep concerns about AI implementation in the hiring process. These concerns revolve around the potential for biased decisions and unfair treatment. This report represented a powerful reminder of the importance of utilizing AI across various industries.
In this blog, we will explore the fascinating and complex ethical ecosystem of Generative AI. From the potential for misuse to the challenges of ensuring fairness and transparency, we explore the critical questions that arise as we stand on the edge of a new era.
Glimpse of Privacy in the Era of Generative AI
As generative AI is evolving, it brings profound privacy concerns. The algorithms capable of developing text, images, and even deepfake videos, raise ethical violations about how personal data is collected, used, and protected.
AI models are trained on vast data sets, which include personal information taken from online sites, social media, and other digital footprints. This data, collected without any user’s consent, is a major data source for algorithms. It enables them to depict human behavior and create highly personalized content. However, the line between convenience and a violation of privacy becomes increasingly blurred.
One of the most pressing privacy issues is misusing user’s personal data. For example, deepfake can create highly resembling but fake videos and audio recordings, posing a threat to personal and public privacy.
Moreover, the opacity of AI models adds another layer to security issues. Usually, users are unaware of how their data is used or the extent to which AI influences the content they see. The lack of transparency makes it difficult to implement ethics in AI.
Ethical Concerns in Generative AI
To help you understand, we have listed the ethical concerns around AI below;
🟠 Misleading Information and Deepfakes
One of the most popular ethical issues is the capability of generating and distributing misinformation. AI algorithms can develop highly realistic but fabricated content including text, images, and videos. Deepfakes can be so convincing that they can be easily confused with the original content. Such generation of misleading information can lead to distributing false information, manipulating public opinion, and damaging the reputation. This can pose serious risks to democratic processes and societal trust.
🟠Bias and Fairness
AI systems can only be unbiased if the data they are trained on is fair. Unfortunately, many datasets include historical and societal biases, which can be evaluated and used by Generative AI. Therefore, the results are biased, which reinforces stereotypes or excludes marginalized groups. To eliminate bias, AI needs efforts to differentiate the unfair and mitigate them in training data and algorithms, promoting inclusivity and equity in AI-generated content.
🟠 Intellectual Property and Ownership
Another ethical dilemma is the authority of content generated by AI. When an AI model generates music, art, or text, defining ownership rights becomes complex. Is it the developers who created the AI, the AI itself, or the users who provided the inputs? The ambiguity poses issues for intellectual property laws and calls for new frameworks to address AI-generated developments.
🟠 Accountability and Transparency
The “black box” characteristic of AI systems makes it overwhelming to understand how they arrive at specific decisions or results. The lack of transparency can lead to difficulties in holding entities accountable for the actions of AI. For example, if an AI-generated recommendation leads to harm, determining responsibility can be problematic. Improving the explainability of AI systems can help create accountability and build users’ trust.
🟠 Compliance Regulations
The capability of AI necessitates responsible use and strong regulations. Developers and other stakeholders are bound to follow ethical principles that protect human well-being, prevent harm, and promote transparency. Regulatory bodies need to establish rules that govern the release of AI models, balancing innovation with societal bias. Public awareness and education about the ethics in AI are vital for responsible utilization.
Ensuring AI Innovation with Responsibility: How We Foster Ethical Guidelines in Our AI Development and Deployment
AI-generated False Political Claim Circulate on Twitter
Social media platforms have become a hotbed for information dissemination, but they have also been exploited for spreading misinformation. A study by The Verge highlights the alarming role of AI content in this context, particularly on Twitter. This report represents how Generative AI can be used to create and share false political claims, leading to significant ethical and societal concerns.
Researchers found that many tweets were created using AI, which eventually spread false information about political candidates, policies, and events. These tweets depicted the language and style of genuine users, making it hard for readers to differentiate between real and fake.
The spread of unwanted and false political claims can negatively influence voter behavior, polarize public opinion, and break trust in political bodies.
Implementing Responsible Generative AI to Avoid Violations
As we stand at the crossroads of technological advancement and ethical considerations, it is important to understand key principles that can be implemented to avoid violations. We have noted down some of these aspects, which you can follow;
🟡 Ethical Practices
Ethics in AI can be maintained initially while designing or developing the system. Developers can actively identify and address biases in the data to avoid perpetuating stereotypes and discrimination.
Organizations should ensure the AI model serves different users and does not disproportionately affect any group. One way this can be done is- involving experts to monitor the development activities. A group of technical experts who are not a part of the development process, along with legal and risk professionals, evaluate the developers’ approach to the system. This strategic approach helps identify gaps or areas of concern in the early stages and addresses the issues immediately.
🟡 Robust Data Practices
To practice responsible Generative AI, it is crucial to have strong data management processes and workflows. Organizations must ensure that data collection and utilization are carried out with explicit user consent. Even the data collected must be stored securely.
While training the AI models, we suggest using high-quality, diverse datasets to train, which helps in reducing biases and improving accuracy.
🟡 Clear Accountability
As we acknowledged earlier, the major challenge of maintaining ethics in AI- is the opacity of accountability. There should be clear establishments of responsibility while developing and implementing the AI models. AI developers should be responsible for the ethical implications of the system, including unintended consequences.
The users deploying Generative AI should understand their ethical obligations and the potential impact of their applications. There should be frequent governmental oversight, including the creation of stringent frameworks and the evaluation of their implementation.
🟡 Continuous Monitoring and Evaluation
AI systems should be frequently analyzed and monitored to ensure ongoing ethical compliance. Conducting regular audits of AI performance to identify and correct biases or inaccuracies can be a beneficial initiative. The developers can incorporate user feedback to improve the system’s performance, fairness, transparency, and usability.
Want to Implement AI? Integrate Gen AI and Ethics with Mindbowser
As we understand the intricate nature of AI and its subsets, the importance of ethical considerations cannot be ignored. It includes addressing the spread of false information, ensuring fairness and transparency. However, with the relevant strategies and practices, the challenges can be overcome effectively, painting the future of AI technologies.
Mindbowser helps you implement your Generative AI efforts ethically, transforming your existing systems and innovating solutions for you. Our data management strategies prioritize user consent, privacy, and security. We maintain the highest data quality standards that result in efficient outputs. We establish industry collaborations and contribute to the development of standards that promote ethical AI practices.
Frequently Asked Questions
How can bias be mitigated in Generative AI?
Bias can be reduced by:
- Starting with high-quality, diverse datasets for training.
- Actively identifying and addressing biases in the data during development.
- Involving a team of experts to monitor development and identify potential biases.
What are some ways to ensure user privacy with Generative AI?
- Obtaining explicit user consent for data collection and usage.
- Storing data securely.
- Developing clear data management practices.
Who is accountable for the ethical implications of Generative AI?
- AI Developers: They are responsible for the system’s design and potential unintended consequences.
- Users Deploying Generative AI: They should understand the ethical implications of their applications.
- Governments: They have a role in creating frameworks and overseeing ethical implementation.
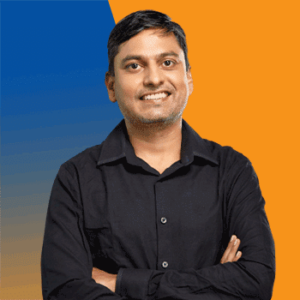
Sandeep Natoo
Head of Emerging Tech
Sandeep Natoo is a seasoned technology professional with a wealth of experience in software development, project management, and leadership. With a strong background in computer science and engineering, Sandeep has demonstrated exceptional proficiency in various domains of technology.
He is an expert in building Java-integrated web applications and Python data analysis stacks. He has been known for translating complex datasets into meaningful insights, and his passion lies in interpreting the data and providing valuable predictions with a good eye for detail.