While machine learning is widely used in the healthcare industry, Generative AI (GenAI) is creating a lot of buzz over its ability to learn from the data sets that it is trained on to create novel content such as images, text, music, videos, and even software codes. Several industries are now bracing to explore its capabilities and looking at opportunities to implement the technology in their applications to bring about innovation breakthroughs.
Morgan Stanley Research indicates a whopping $6 Trillion worth of opportunity in Generative AI that can bring a transformative change in how we do business today. It wouldn’t be wrong to say that Generative AI is here to stay rather than being a mere passing trend as it has the potential to raise global GDP by 7%, as per the Goldman Sachs Research report.
The healthcare industry is not far behind in catching up with this trend, as a McKinsey report suggests that investment in the healthcare sector has the potential to generate approximately $1 trillion to $1.5 trillion through 2027. Leaders in the healthcare industry are keen to embrace this new change as they seek new ways to utilize Generative AI through innovative use cases that have created both excitement and curiosity among healthcare professionals.
In this article, we look at some of the prominent use cases of Generative AI in the healthcare industry that can improve overall patient care and help in developing solutions that aim to bring positive outcomes. We also look at some of the challenges of using Generative AI and what can be done to overcome them.
Top 12 Use Cases of Generative AI in the Healthcare Industry
Although there are numerous use cases of Generative AI in the healthcare industry that can enhance efficiency, accuracy, and patient care, some of the prominent use cases provided are:
1. Digital Self-Service Culture
Self-service culture provides patients access to healthcare information such as records, lab reports, and medical notes through a digital device. This culture of getting access to information at the fingertips coupled with Generative AI can help in creating AI-powered virtual assistants and chatbots that can use their intelligence to provide relevant suggestions to patients.
Virtual assistants and chatbots can help patients improve overall patient engagement and allow them to play a very active role in managing their health by:
🔹Managing appointment scheduling, sending medication reminders, addressing general health inquiries, and offering tailored health recommendations based on an individual’s medical history and current health status.
🔹Providing information and assistance around the clock, handling routine administrative tasks, and allowing healthcare professionals to focus more on complex and specialized patient care.
🔹Delivering mental health support by engaging in conversations, providing coping strategies, and identifying individuals in crisis who may need immediate intervention.
2. Customized Care & Guided Diagnosis
The vast amount of healthcare data is fragmented across medical records and family history which can be easily analyzed using Generative AI technology to summarize relevant information. This information can be used for curating customized care plans for each individual patient based on their unique requirements. These Generative AI-based systems can:
🔹Identify patterns within the data and generate treatment recommendations that can help healthcare providers make informed decisions and optimize the effectiveness of healthcare interventions.
🔹Create patient-specific health content in the form of informative materials and educational resources to help patients understand their conditions and adhere to prescribed therapies. Healthcare providers can then choose the most effective healthcare plan with minimum cost.
🔹Analyze medical images, lab reports, and health records to provide predictive analysis for early diagnosis and detection of health issues. Healthcare professionals can make timely and appropriate treatment plans for patients leading to better outcomes.
3. Connecting Dots of Population Health Management
Healthcare organizations and policymakers can use Generative AI to analyze large amounts of data that can be used to identify healthcare trends in the population and allocate appropriate resources based on the information. This leads to an overall improvement in the well-being of the population and effective outcomes. Generative AI platforms can:
🔹Analyze data to identify people with high-risk health conditions and use predictive analysis to implement appropriate measures to prevent diseases.
🔹Analyze the health behaviors among people on various parameters such as diet, exercise, or medication to identify patterns that can be further used to create personalized healthcare programs and plans. This motivates people to adopt healthier lifestyles and make appropriate choices that lead to better health and quality of life.
🔹Simulate and predict disease outbreaks by analyzing various parameters such as historical data, environmental factors, and population movements. This helps health agencies to ensure better preparedness for handling such outbreaks and take appropriate measures to minimize the impact of the pandemics.
4. Medical Research & Stimulations
Extensive medical literature can be easily analyzed by using Generative AI to identify topics of research which can be further used by researchers to identify and target new research and generate hypotheses. The algorithm can process and analyze vast amounts of biomedical data such as genetic and clinical data, to identify patterns, potential drug targets, and disease markers. The researchers can then use this information to:
🔹Uncover hidden insights easily within complex datasets that otherwise can be challenging and time-consuming using a manual approach.
🔹Simulate biological processes, disease progression, and drug interactions in virtual environments. Researchers can use these simulations to test hypotheses, explore various treatment strategies, and gain a deeper understanding of complex diseases.
🔹Facilitate personalization of medicine by analyzing an individual’s genetic makeup and health history and generate simulations of how diseases may progress in that specific person. This enables doctors to tailor treatments and interventions to each patient’s unique characteristics, improving treatment outcomes.
5. Improved Operational Works
The operational aspect of healthcare facilities can be achieved easily by using Generative AI to enhance efficiency and allocate appropriate resources. The AI models can analyze data to predict patient demand and further help in allocating healthcare staff to handle patients effectively and reduce burden. Conducting analysis of past usage data through Generative AI can:
🔹Forecast the demand for medical supplies and pharmaceuticals, optimizing inventory levels and reducing waste. This ensures that healthcare facilities have the right medications and equipment on hand when needed, avoiding shortages or overstock situations.
🔹Analyze patient admission and discharge data, along with real-time patient information, to optimize bed allocation. This ensures effective allocation of beds to patients, reducing wait times and improving the patient experience.
🔹Predict staffing needs based on patient volumes and assist healthcare facilities to ensure they have the right number of healthcare professionals on hand to provide high-quality care.
🔹Analyze data from medical equipment and systems to predict when maintenance is needed. This proactive approach helps prevent equipment breakdowns, reduces downtime, and ensures that critical medical equipment is always available for patient care.
6. Medical Notetaking
Healthcare professionals spend a considerable amount of time adding or updating medical notes in the Electronic Health Record (EHR) system. Generative AI can ease this process by:
🔹Capturing notes, converting handwritten notes into digital records, and summarizing lengthy medical documents. This not only saves healthcare providers valuable time but also ensures accuracy and consistency in documentation.
🔹Organizing and structuring the medical notes by defining appropriate categories and tags for notes. This helps to retrieve specific data when needed and streamlines the retrieval of relevant patient information during follow-up visits and consultations.
🔹Ensuring compliance with healthcare regulations and standards by flagging any incomplete or missing information in medical notes, reducing the risk of errors and omissions that could impact patient care and billing accuracy.
🔹Automating and enhancing medical notetaking empowers healthcare professionals to focus more on patient care and less on administrative tasks.
Want to Leverage The Power of Generative AI for Your Business?
7. Early Diagnosis of Diseases
Early detection of diseases is important especially in the case of rare diseases to provide effective and timely treatment and improve positive outcomes. Analyzing the vast amount of data set in the form of electronic health records (EHRs), medical imaging, and genetic information enables Generative AI to:
🔹Identify subtle patterns and anomalies that might signal the presence of diseases in their early stages. For example, CT scans can be analyzed to detect the possibility of lung cancer or stroke. This helps in early diagnosis by predicting disease risk factors and adopting prevention strategies.
🔹Provide high accuracy in early detection of disease which might be often missed by human eyes. Integrating clinical data with genetic information and assessing lifestyle factors using Generative AI can help to create a holistic view of a patient’s health, allowing for more accurate and personalized early diagnosis.
🔹Allow healthcare professionals to make informed decisions by flagging potential risks during routine visits. For example, it can analyze a patient’s historical data and suggest additional tests or screenings based on risk factors or emerging trends in the data.
8. Improved Clinical Decision
By analyzing and processing vast volumes of medical data, including patient records, diagnostic images, lab results, and scientific literature, Generative AI can extract critical insights that can be used by healthcare professionals to support their clinical judgments. Comparing the analyzed data to a vast database of similar cases, Generative AI can help to:
🔹Identify diseases and suggest potential diagnoses, reducing the potential risk of misdiagnosis.
🔹Provide suggested evidence-based treatment options taking into account various factors into consideration such as medical history, genetics, and responses to previous therapies. These suggestive treatments are tailored to meet the requirements of individual patients, optimizing their outcomes.
🔹Provide real-time decision support in emergency situations and help prioritize patients based on the severity of their conditions, improving the allocation of limited resources.
9. Drug Discovery & Development
Traditional drug discovery is time-consuming and costly, often taking years to identify viable candidates. Generative AI can rapidly generate and evaluate thousands of potential drug compounds in silico, significantly shortening the discovery phase and helping to:
🔹Improve the success rate and reduce the overall cost required for drug development.
🔹Optimize existing drugs by analyzing chemical structures and properties and suggest modifications to enhance a drug’s efficacy or reduce side effects, leading to the development of improved versions of existing medications.
🔹Facilitate target identification by analyzing biological data to identify new drug targets and pathways involved in diseases, providing opportunities for novel drug development.
🔹Enhance the process of virtual screening of compounds, allowing researchers to identify the most promising drug candidates from vast chemical libraries. This reduces the need for extensive laboratory testing, saving time and resources.
10. Medical Devices Submissions
Securing approvals from regulatory bodies such as the Food and Drug Authority (FDA) for medical devices is often complex, time-consuming, and costly. The medical submission process can take a long time up to several years depending upon the complexity of the devices. Generative AI can assist medical device manufacturers in this complex process by:
🔹Analyzing existing regulatory documents and guidelines to generate reports and documentation that adhere to specific regulatory requirements, reducing the potential for errors and speeding up the approval process.
🔹Contributing to post-market surveillance by analyzing real-world data from medical devices already in use. It can detect potential safety issues or adverse events, enabling quicker responses to emerging risks and ensuring patient safety.
🔹Creating virtual models and simulations of medical devices to allow manufacturers to test their devices in various scenarios without the need for physical prototypes. This can reduce development costs and accelerate the innovation cycle.
11. Medical Device Predictive Maintenance
Generative AI can analyze and process data from various sensors and monitoring systems integrated into medical devices to predict when maintenance is required. This proactive approach helps healthcare facilities perform maintenance tasks effectively and prevent equipment downtime. Generative AI can help to:
🔹Detect deviations in device performance to notify the maintenance teams in advance, ensuring timely repairs or replacements, and reducing the risk of equipment failure during critical medical procedures.
🔹Tailor maintenance plans based on the actual condition of the equipment instead of relying on fixed maintenance intervals. This saves time and resources while ensuring equipment reliability. By addressing issues early, wear and tear can be minimized, ultimately reducing the frequency of costly replacements and lowering healthcare operational costs.
🔹Contribute to patient safety by ensuring that medical devices are operating within specified parameters, reducing the risk of malfunctions that could harm patients or compromise the quality of care.
12. Faster and Smoother Interpretation of Unstructured Medical Data
The healthcare industry generates large amounts of unstructured data that is often scattered across different systems and is often difficult to store. This unstructured data can be processed by using Generative AI and transformed into structured and standardized information. Physicians and healthcare providers can input unstructured patient data into Generative AI systems to:
🔹Extract and organize relevant information, providing a comprehensive patient profile for quicker decision-making.
🔹Assist in the review of medical literature to analyze and summarize research papers, clinical trials, and case studies, allowing healthcare professionals to stay updated on the latest medical advancements and evidence-based practices.
🔹Extract diagnostic and procedural information automatically from clinical notes, reducing the administrative burden on medical coders and ensuring accurate and timely reimbursement for healthcare services.
🔹Contribute to clinical research by facilitating data extraction from patient records for research purposes, streamlining the recruitment of participants for clinical trials, and aiding in the identification of suitable candidates for specific studies.
Challenges GenAI Poses for the Healthcare Industry
🔸Biased Approach & Discrimination
The training data fed to the Generative AI models could include bias that might be passed on to the output generated by these models. In other industries, these can often lead to inefficiencies but in healthcare, this biased approach can have serious implications for a patient’s health.
The training data can include racial bias, gender bias, or socioeconomic bias that can lead to incorrect decisions and discrimination among patients. For instance, due to the biased approach, Generative AI may inadvertently discriminate against certain demographic groups by providing inaccurate or unfair healthcare recommendations.
Involving AI developers with multiple backgrounds to train the model, curating and using high-quality data sources from reliable sources, and ensuring adherence to the regulatory framework can reduce the bias and generate positive, unbiased outcomes.
🔸Lack of Transparency
Due to the complexities of the Generative AI models, it is challenging for healthcare professionals to understand how the AI arrives at specific recommendations or diagnoses. This issue can lead to a lack of trust among healthcare professionals as they might be hesitant to rely on AI-generated insights.
Complex algorithms, lack of visibility in selecting appropriate data sources and data sets, and bias in training data can lead to transparency issues in decisions made by AI.
Implementing explainability and interpretability features in Generative AI models allows healthcare professionals to grasp the rationale behind AI-generated decisions. Involving healthcare professionals in the loop and ensuring consistency in decision-making can help to eliminate transparency issues in generated insights.
🔸Intellectual Property & Copyright Infringements
Generative AI models are often trained on large datasets that can include copyrighted medical information, research papers, or proprietary healthcare data. When these models generate content, there’s a potential risk that they might inadvertently produce outputs that replicate copyrighted materials, leading to copyright infringement issues.
To avoid legal complications, healthcare professionals and AI developers must ensure that generated content respects copyright and intellectual property laws.
Additionally, it is difficult to determine the owner of the information created by AI and can potentially create problems in deciding who can use this newly generated information and cause copyright violations. To determine the ownership of AI-generated information it is important to implement robust copyright laws and frameworks that address authorship and intellectual property protection in the context of AI-generated content.
🔸Privacy & Security
Healthcare data in the form of personal medical records, patient histories, and even genomic information is highly sensitive and regulated by data regulation policies and frameworks. When this data is processed by using Generative AI applications, there is a substantial risk of breaches or unauthorized access that could lead to the leaking of confidential information.
Additionally, in case of cyber-attacks, there are chances of unauthorized modifications of AI models to produce incorrect or harmful results. In healthcare, this could have life-threatening consequences if treatment recommendations or diagnoses are tampered with.
To address these challenges, healthcare organizations must implement stringent security measures, including robust encryption, access controls, and regular security audits. Additionally, there’s a need for ethical guidelines and regulatory frameworks to ensure the responsible and secure deployment of Generative AI in healthcare, safeguarding patient privacy and data integrity.
🔸False Results
Poor quality data used for training the GenAI models can lead to producing inaccurate or misleading outputs that can have a huge impact on patient health and well-being. For instance, a false diagnosis generated by GenAI could lead to unnecessary treatments, emotional distress for patients, or delayed diagnosis of serious conditions.
Similarly, false predictions regarding disease progression or treatment responses could lead to suboptimal patient care.
To address this challenge, continuous validation and testing of GenAI models are essential. Healthcare professionals must remain vigilant and exercise clinical judgment when interpreting AI-generated results.
🔸Misuse or Overreliance
Healthcare providers might not use the GenAI capabilities to their fullest potential due to reluctance or lack of trust. This might lead to underutilization or missed opportunities for more accurate diagnoses or treatment recommendations, impacting patient care. On the other end, they might over-rely on AI-generated insights and not use their own clinical judgment or neglect the critical human factor in healthcare.
Providing training and education to healthcare professionals about the strengths and limitations of AI is essential. Additionally, implementing guidelines and best practices for the responsible use of AI tools in clinical settings can help mitigate the risk of misuse or overreliance. Striking the right balance between AI support and human expertise is key to optimizing patient care while avoiding undue dependence on AI in healthcare decision-making.
How Mindbowser Can Help You Integrate Generative AI in Your Healthcare System?
Generative AI has a lot of potential to bring about a positive transformative change in how we perceive healthcare today. The journey to adopting Generative AI in healthcare requires close collaboration with a technological partner having expertise in implementing world-class solutions with quality and precision, in-depth knowledge of healthcare regulations, and a strong commitment to patient privacy and data security.
Mindbowser offers a comprehensive solution for seamlessly integrating Generative AI into your healthcare system. Our expertise in AI-driven healthcare solutions ensures a smooth integration process. We provide tailored models and algorithms that can assist in tasks such as medical image analysis, patient data processing, and predictive analytics.
Our team of AI specialists working closely with your organization can help you customize the implementation, ensuring compliance with healthcare regulations and optimizing the use of Generative AI to enhance patient care, streamline operations, and improve overall healthcare outcomes.
Frequently Asked Questions
What is a real-life example of generative AI?
Generative AI is used in various industries such as healthcare, education, marketing, and tourism. Applications such as Genei for summarizing PDFs and websites and Synthesia for creating engaging videos from text for learning are some examples of Generative AI in the education field. Similarly, Jasper Campaigns and RAD AI are used for end-to-end marketing campaigns.
What problems can generative AI solve?
GenAI can help to solve numerous problems by:
- Creating human-like content in the form of text or images that can be used in content creation, marketing, or even arts and design.
- Helping healthcare professionals in diagnosing diseases, analyzing images such as CT scans or X-rays, predicting patient outcomes, and improving overall patient care.
- Translating content in real-time to break communication barriers and contribute to seamless communication.
- Accelerating drug discovery by simulating molecular interactions, suggesting potential drug candidates, and analyzing vast biomedical datasets.
How to use generative AI in daily life?
Generative AI can be integrated into daily life in various ways:
- Writing articles, generating creative content, or composing music, saving time and sparking creativity.
- Virtual assistants like Siri, Alexa, or Google Assistant use generative AI to answer questions, control smart devices, and assist with tasks like setting reminders and managing your schedule.
- Streaming platforms use generative AI to recommend movies, music, and TV shows tailored to your preferences, enhancing your entertainment experience.
- Some health apps utilize generative AI to provide personalized health recommendations and track fitness progress.
- Many customer service and support chatbots employ generative AI to provide instant responses to inquiries or troubleshoot issues.
- Generative AI tools can help artists and designers create digital art, designs, and animations, even generating new design ideas.
What are the most popular generative AI models?
What is an example of generative AI in healthcare?
Generative AI can be integrated into wearable devices to collect and analyze the data. This helps to customize the healthcare plans as per the requirements of the users and enables them to make informed choices for their well-being. A metabolic health company, January.ai, is harnessing the power of generative AI to focus on monitoring glucose levels. Using this technology, January.ai can estimate and anticipate how individuals will respond to different foods in terms of their glucose levels, even when they are not wearing a continuous glucose monitor (CGM). This empowers individuals to make informed and intelligent decisions when it comes to their dietary choices.
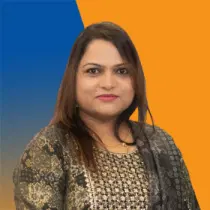
Manisha Khadge
CTO, Mindbowser
Manisha Khadge, recognized as one of Asia’s 100 power leaders, brings to the table nearly two decades of experience in the IT products and services sector.
She’s skilled at boosting healthcare software sales worldwide, creating effective strategies that increase brand recognition and generate substantial revenue growth.