Healthcare businesses are embracing innovative technologies to completely transform their operations. One such technology that is getting its roots stacked with significant impact is Large Language Models (LLMs). These advanced AI models have the potential to understand and generate human-like language on a large scale. Empowered by machine learning, LLMs are revolutionizing communication and driving remarkable advancements in the healthcare industry.
The integration of LLMs in the healthcare landscape is the greatest technological advancement. From improving data analysis and decision-making processes to transforming patient engagement and administrative efficiency, LLMs have the potential to bring about a paradigm shift in how healthcare organizations operate.
As we delve into this blog, we will explore the most crucial aspects that healthcare businesses need to know about LLMs and how they can leverage LLMs advancements for exceptional outcomes.
Key Advantages of Implementing LLMs in Healthcare
LLMs bring multiple advantages to healthcare, revolutionizing traditional practices and paving the way for improved efficiency and patient-centric healthcare. Together let’s explore some of the benefits of integrating LLMs in healthcare;
Improved Decision-making Processes
One of the advantages of implementing LLMs in healthcare is the improvement in decision-making processes. LLMs are well-equipped with large datasets that help healthcare providers in analyzing complex medical data, research articles, and patient histories. This helps in the diagnostic phase and ensures the treatment plans are more informed and tailored to individual patient needs.
Enhanced Patient Experience
Patient engagement and satisfaction are central to the success of any healthcare organization. LLMs elevate the patient experience by facilitating improved communication and information dissemination. These models can be deployed in different touchpoints, from virtual health assistants that offer timely medical information to chatbots providing support for appointment scheduling. Natural Language Processing capabilities, create a more accessible and personalized interaction between healthcare providers and patients.
Streamlined Administrative Tasks
The administrative tasks of healthcare professionals can be overwhelming, impacting operational efficiency. LLMs come to the rescue as an efficient solution to automate and streamline various administrative tasks. From transcribing medical notes to managing appointment schedules and handling daily inquiries, models can significantly reduce the admin workload.
Potential Cost Savings
LLMs offer the prospect of substantial cost savings for healthcare organizations. It contributes to operational efficiency, translating into reduced operational costs. The capabilities for more accurate diagnoses and treatment plans hold the promise of preventing costly medical errors. As healthcare providers navigate financial constraints, the implementation of LLMs emerges as a strategic investment with the potential for long-term economic benefits.
Scalable Healthcare Practices
The rapid evolution of healthcare technologies necessitates a forward-thinking approach to ensure organizations remain relevant and adaptable. LLMs, with their capacity to evolve and learn continuously, contribute to scalable healthcare practices. By staying ahead of the latest medical research, treatment protocols, and industry trends, language models empower healthcare organizations to adapt swiftly to changes.
Ready to Transform Your Healthcare Approach?
Common Challenges Faced While Implementing LLMs in Healthcare
As healthcare organizations and providers are utilizing the capabilities of Large Language Models, it’s important to look out and address the potential concerns and challenges associated with its integration. Understanding and proactively mitigating these issues is important to ensure a seamless transition into advanced healthcare solutions.
Data Privacy and Security
When it comes to utilizing LLMs in healthcare, one of the major issues is the privacy and security of patient information. Given the sensitive nature of healthcare data, it is important to address these concerns effectively.
To ensure privacy and security, strong encryption protocols should be implemented to protect patient data while storing and transmitting. You can utilize advanced encryption techniques that render the data unreadable to unauthorized individuals.
Healthcare providers or organizations should adhere to regulatory standards such as HIPAA. The regulatory act provides a detailed framework for data protection, ensuring patient data is secure. Every stakeholder in healthcare can address any issues or potential breaches by continuously monitoring and improving the security protocols.
Ethical Considerations
LLMs in healthcare expose a series of ethical questions around decision-making, accountability, and potential biases embedded within these models. It is important to address these issues actively to ensure responsible and equitable use of LLMs in the healthcare industry.
To address these ethical challenges, it is important to establish clear guidelines for the development of LLMs in healthcare. These guidelines act as ethical considerations, ensuring that the models are designed and used in a way that upholds patient care and respects privacy rights.
Implementing transparency while utilizing LLMs in decision-making processes. Healthcare organizations can maintain accountability and build trust with other stakeholders. Clear documentation of “How LLMs are implemented in their decision-making” including the aspects considered and any limitations, helps in maintaining complete transparency.
A strong commitment to audit and assessing LLMs for any biases is important. Bias within these models can minimize inequalities and have significant consequences for patient care.
Integration with Existing Systems
As the implementation of LLMs will be new, many issues and complexities will arise with the existing healthcare system. It requires careful planning and strategic measures to effectively address these challenges.
Conduct compatibility assessments before implementing LLMs into your existing systems. The assessment will offer a visibility of any bottlenecks in integrations of language models. Get effective assistance from experts who can develop seamless integration strategies that reduce disruptions to established workflows. Their expertise ensures technical considerations are solved, resulting in efficient and effective incorporation.
Training and Development of Healthcare Professionals
Healthcare professionals may face challenges in adapting to and effectively using LLMs, requiring additional training and development.
Organizations can implement multiple training programs to make healthcare professionals understand and be familiar with LLM capabilities and their usage. Provide ongoing support and resources for continuing education. Such activities keep healthcare staff updated on the latest advancements and their implications.
You can even collaborate with experts, who can guide and provide proper information about integrating AI and LLMs into healthcare.
Patient Trust and Acceptance
Another challenge in hand is to build trust among patients regarding the utilization of LLMs in healthcare and ensure their acceptance of AI-driven processes.
As we discussed earlier, ensuring transparent communication with patients is crucial. Provide clear information about the benefits and potential risks which will develop a proper understanding and trust in the solution implemented.
A second utmost important aspect is giving patients control over the extent of LLM involvement in their healthcare treatment or services. Implementing consent mechanisms ensures that the patients can make correct decisions about their treatment options.
Educating patients about the positive impact of LLMs on their overall care is important to build acceptance. Awareness campaigns can help solve any misconceptions, increase knowledge, and promote the benefits of AI-driven processes.
Exploring LLMs in Healthcare Landscape
OpenAI recently conducted tests to examine the performance of its latest model, GPT-4 in the healthcare industry. There were impressive results found regarding the performance of GPT-4 in medical applications. The tests included the MultiMedQA dataset and real questions from the US Medical License Exam. It performed better than the older GPT-3.5 model and other AI models which were specifically designed for medical inquiries. GPT-4 demonstrated enhanced self-awareness, accurately discerning the confidence of its answers.
The introduction of pre-trained models for medical applications has brought advancements in healthcare delivery. Examples such as BioBERT, ClinicalBERT, BlueBERT, and BioGPT have changed AI implementations in the medical field. The biomedical corpora specialization in BioBERT enables me to excel in comprehending important medical texts and terminology.
The model has determined its capabilities in various tasks such as disease prediction, drug-drug interaction analysis, and biomedical named entity recognition. Building upon BioBERT, ClinicalBERT undergoes additional fine-tuning using clinical notes. This improves the ability to process clinical nature language and interpret patient’s EHRs.
These pre-trained models have proved as a more effective utilization of AI in healthcare, empowering medical professionals with advanced tools for diagnosis, treatment planning, and medical research.
LLMs in Electronic Health Record Analysis
Healthcare LLMs improve clinical documentation accuracy and efficiency in EHR systems by automatically reviewing and analyzing records, identifying missing data, and suggesting any additions if required. LLMs act as a valuable tool for clinical decision support, offering evidence-based recommendations and alerts about drug interactions.
One of the greatest examples is an LLM-powered solution by JohnSnow Labs, which analyzes EHR data and identifies medication non-adherence patterns. It eventually improved patient outcomes and care delivery.
LLMs in Clinical Text Analysis
The application of LLMs in Clinical Text Analysis helps in extracting insights from unstructured clinical texts like EHRs and radiology reports. They can automatically extract key data with efficient indexing and retrieval. LLMs can adversely monitor by analyzing text data and identifying potential safety concerns. These models contribute to timely diagnosis, improve patient safety, and support pharmacovigilance efforts.
LLMs in Drug Discovery and Development
Medical LLMs play a major role in drug discovery and development by analyzing biomedical literature, scientific papers, and clinical data. They identify potential drug targets, disease mechanisms, and genetic aspects of specific diseases. Such LLMs help researchers uncover novel drug targets and validate their efficacy. Medical LLMs contribute largely to drug repurposing by finding new applications for existing drugs, reducing the time and costs involved in developing new treatments.
LLMs in Medical Image Analysis
LLMs associated with natural language processing, extend text processing capabilities. They can also be applied to different tasks, including medical image analysis. LLMs can be utilized to analyze and extract valuable information from medical images, such as X-rays, MRIs, CT scans, and other diagnostic images.
Healthcare organizations can train LLMs to recognize patterns and anomalies in medical images. This can be particularly valuable in detecting diseases, tumors, or abnormalities that may not be immediately apparent to human observers.
How Mindbowser Can Help You with Integrating LLMs in the Healthcare Landscape?
In this dynamic intersection of healthcare and advanced technology, the integration of LLMs has transformed patient care, medical research, and organizational efficiency. As we conclude our exploration of LLMs in the healthcare landscape, it becomes clear that these advanced language models are not just tools but catalysts for positive change.
From improving decision-making processes and enhancing patient experiences to streamlining administrative tasks, our experts at Mindbowser help you leverage the advantages that reshape traditional healthcare delivery. We promise to achieve cost savings and scalable practices while embracing these technological innovations.
Our exploration has also delved into the intricacies of LLMs, representing their applications in medical image analysis, clinical decision support, and even the potential to contribute to drug discovery. We will help you understand and bridge the gaps between different modalities of medical information.
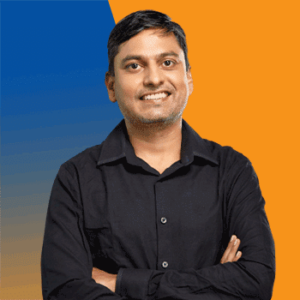
Sandeep Natoo
Head of Emerging Tech
Sandeep Natoo is a seasoned technology professional with a wealth of experience in software development, project management, and leadership. With a strong background in computer science and engineering, Sandeep has demonstrated exceptional proficiency in various domains of technology.
He is an expert in building Java-integrated web applications and Python data analysis stacks. He has been known for translating complex datasets into meaningful insights, and his passion lies in interpreting the data and providing valuable predictions with a good eye for detail.